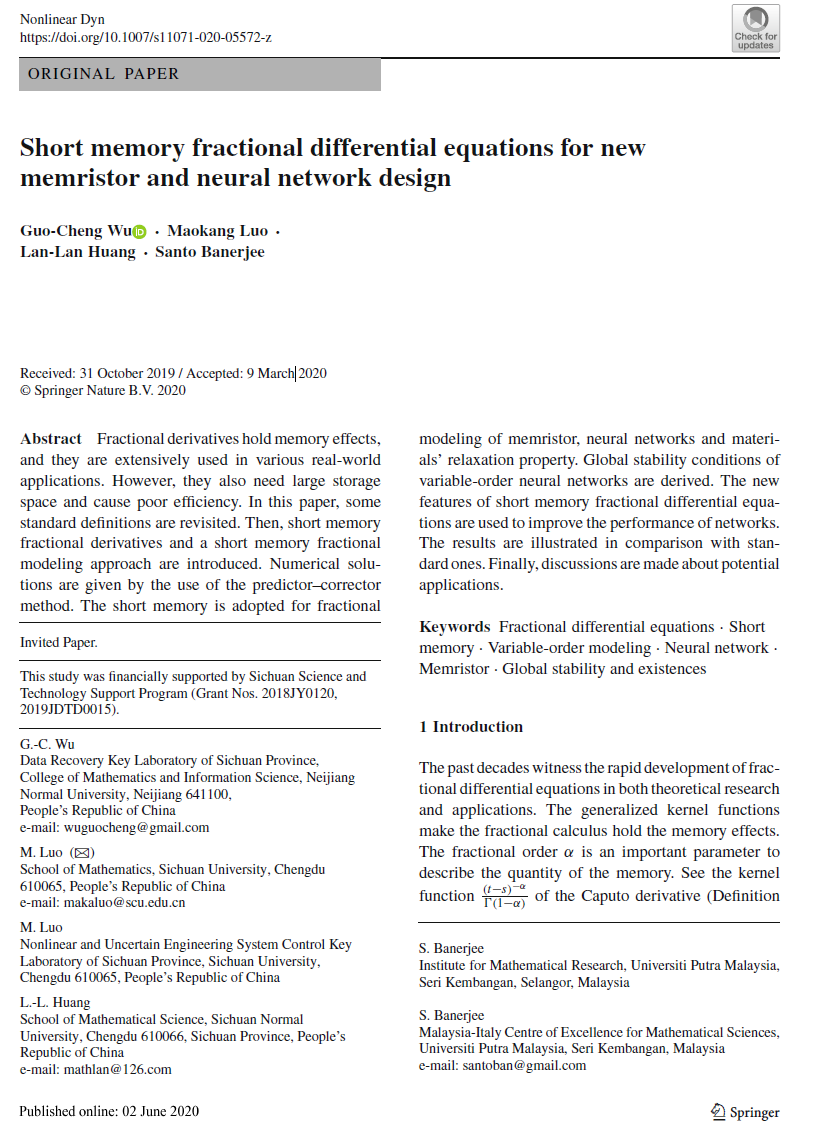
2020年6月2日,数据恢复四川省重点实验室复杂网络与保密通信方向吴国成老师团队在国际期刊《Nonlinear Dynamics 》(IF:4.604,SCI二区)发表题为《Short memory fractional differential equations for new memristor and neural network design》的研究论文。
Abstract:Fractional derivatives holdmemory effects, and they are extensively used in various real-world applications. However, they also need large storage space and cause poor efficiency. In this paper, some standard definitions are revisited. Then, short memory fractional derivatives and a short memory fractional modeling approach are introduced. Numerical solutions are given by the use of the predictor–corrector method. The short memory is adopted for fractional modeling of memristor, neural networks and materials’ relaxation property. Global stability conditions of variable-order neural networks are derived. The new features of short memory fractional differential equations are used to improve the performance of networks. The results are illustrated in comparison with standard ones. Finally, discussions aremade about potential applications.